REASERCH RESULT
研究論文のご紹介です。
-
アンサンブル予報を用いた確率的風予測と巡航速度誘導への適用
2023 August
-
Large-Eddy Simulation of Wake Vortices at Tokyo/Haneda International Airport
2023 April
-
Emulating Rainfall–Runoff-Inundation Model Using Deep Neural Network with Dimensionality Reduction
2023 January
-
デジタルツインとデータ同化
2022 December
-
羽田空港の格納庫後流中を飛行する着陸機の安全性の研究
2020 April
-
Real-time estimation of airflow vector based on lidar observations for preview control
2020 December
-
回帰学習器のアンサンブル学習による降雨洪水氾濫モデル・エミュレータ
2020 August
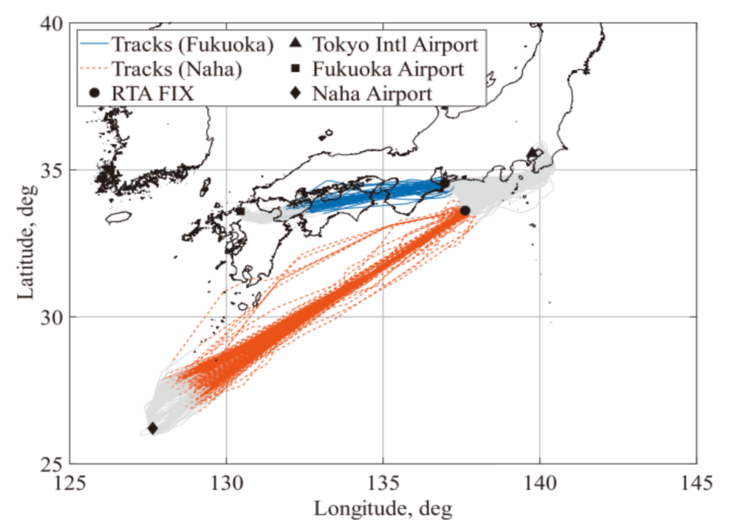
アンサンブル予報を用いた確率的風予測と巡航速度誘導への適用
- 著者・発表者
- 松野 賀宣(国立研究開発法人 宇宙航空研究開発機構)
菊地 亮太(DoerResearch株式会社, 名古屋大学)
- 掲載・発表場所
- 日本航空宇宙学会誌
- 掲載・発表日
- 2023年8月5日
航空機運航において、到着予定時刻(Estimated Time of Arrival: ETA)の予測精度は風予測誤差に大きく影響される。本稿では、風予測誤差の影響を緩和し、ETAの予測精度を向上させるため、確率的風予測手法を提案し、巡航速度誘導則に適用する。確率的風予測では、多数の数値気象予報を行うアンサンブル予報を用いて、風を確率的に推定することで、ETAを高精度に予測する。そして、速度誘導則では、所定の許容範囲内で目標の到着時刻を満たす最適速度を決定する。本稿では、数値シミュレーションにより、確率的風予測を適用した速度誘導則の性能及び有効性を評価する。確率的風予測を適用することで、典型的な飛行管理装置に比べ、ETA予測精度が向上し、目標の到着時刻を満たすための不必要な速度変更回数を低減することができるため、航空交通管制による軌道予測性の向上や燃料消費量の削減効果が期待される。
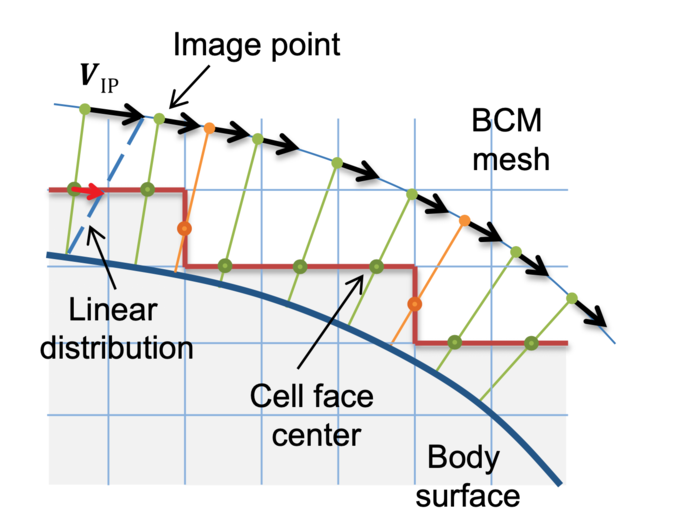
Large-Eddy Simulation of Wake Vortices at Tokyo/Haneda International Airport
- 著者・発表者
- Takashi Misaka(National Institute of Advanced Industrial Science and Technology)
Ryoichi Yoshimura(Tohoku University)
Shigeru Obayashi(Tohoku University)
Ryota Kikuchi(Japan Aerospace Exploration Agency)
- 掲載・発表場所
- Journal of Aircraft
- 掲載・発表日
- 2023年4月18日
A large-eddy simulation (LES) of wake vortices in ground effect is conducted under the influence of airport hangar buildings to investigate the airport-specific behavior of wake vortices. We consider a landing at Tokyo/Haneda International Airport in Japan, where a wake of hangar buildings covers a landing path and touchdown zone under particular wind conditions. We combine an LES code and the Weather Research and Forecasting numerical weather prediction model to retrieve realistic wind conditions around the airport. The behavior of wake vortices is then investigated in the retrieved wind field using the adaptive mesh capability of the LES code for capturing the tiny vortex core of the wake vortex. At the crosswind on the order of a vortex descent speed, the hangar wake reduces the crosswind near the runway, enhancing the windward vortex’s rebound. The effect is alleviated by doubling the crosswind to twice the vortex descent speed. On the other hand, the wind fluctuations caused by the hangar buildings only slightly affect the wake vortex decay.
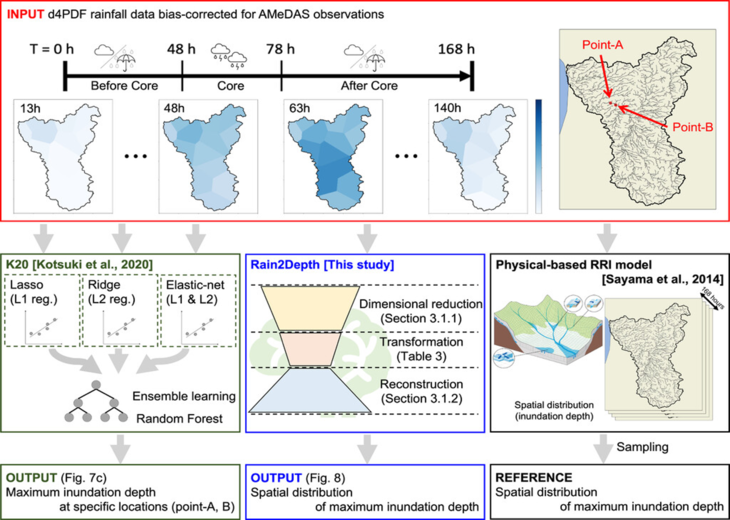
Emulating Rainfall–Runoff-Inundation Model Using Deep Neural Network with Dimensionality Reduction
- 著者・発表者
- Momoi Masahiro(GRASP SAS, DoerResearch, Inc.)
Kotsuki Shunji(Chiba University, RIKEN Center for Computational Science, Japan Science and Technology Agency)
Kikuchi Ryota(Kyoto University, DoerResearch, Inc.)
Watanabe Satoshi(Kyoto University)
Yamada Masafumi(Kyoto University)
Abe Shiori(Mitsui Consultants Co., Ltd.)
- 掲載・発表場所
- Artificial Intelligence for the Earth Systems
- 掲載・発表日
- 2023年1月1日
Predicting the spatial distribution of maximum inundation depth (depth-MAP) is important for the mitigation of hydrological disasters induced by extreme precipitation. However, physics-based rainfall–runoff-inundation (RRI) models, which are used operationally to predict hydrological disasters in Japan, require massive computational resources for numerical simulations. Here, we aimed at developing a computationally inexpensive deep learning model (Rain2Depth) that emulates an RRI model. Our study focused on the Omono River (Akita Prefecture, Japan) and predicted the depth-MAP from spatial and temporal rainfall data for individual events. Rain2Depth was developed based on a convolutional neural network (CNN) and predicts depth-MAP from 7-day successive hourly rainfall at 13 rain gauge stations in the basin. For training the Rain2Depth, we simulated the depth-MAP by the RRI model forced by 50 ensembles of 30-yr data from large-ensemble weather/climate predictions. Instead of using the input and output data directly, we extracted important features from input and output data with two dimensionality reduction techniques [principal component analysis (PCA) and the CNN approach] prior to training the network. This dimensionality reduction aimed to avoid overfitting caused by insufficient training data. The nonlinear CNN approach was superior to the linear PCA for extracting features. Finally, the Rain2Depth architecture was built by connecting the extracted features between input and output data through a neural network. Rain2Depth-based predictions were more accurate than predictions from our previous model (K20), which used ensemble learning of multiple regularized regressions for a specific station. Whereas the K20 can predict maximum inundation depth only at stations, our study achieved depth-MAP prediction by training only the single model Rain2Depth.
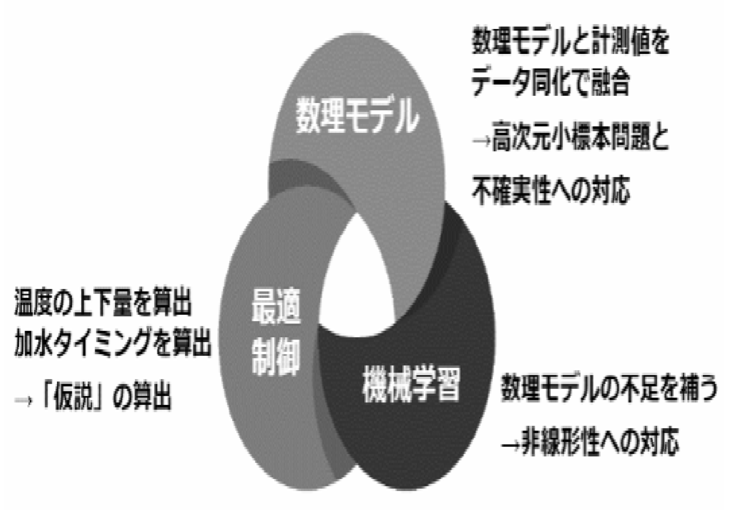
デジタルツインとデータ同化み
- 著者・発表者
- 三坂 孝志(産業技術総合研究所)
菊地 亮太(京都大学, DoerResearch株式会社)
- 掲載・発表場所
- CMD Newsletters(日本機械学会)
- 掲載・発表日
- 2022年12月8日
「デジタルツイン」はバズワードのように感じられるが、デジタルツインという言葉が使われ始めた2011年頃の航空分野の論文では、航空機デジタルツインの実現シナリオも示されている。出版から10年以上経過して情報通信技術が進歩した現在ではこれを少し拡大解釈して、デジタルツインは「現実とリアルタイムに同期されるデジタル空間上のシミュレーションモデル」と理解できる。筆者らが利用しているデータ同化は、数値シミュレーションにおける不確かな条件・パラメータをベイズ統計により計測デー タに基づき推定する手法でり、気象予測などで応用されて広がっている。デジタルツインとの関連は、デジタル空間上のモデルを現実と同期させる手法として利用できる。そのような文脈でデータ同化の活用事例を2例ほど紹介する。
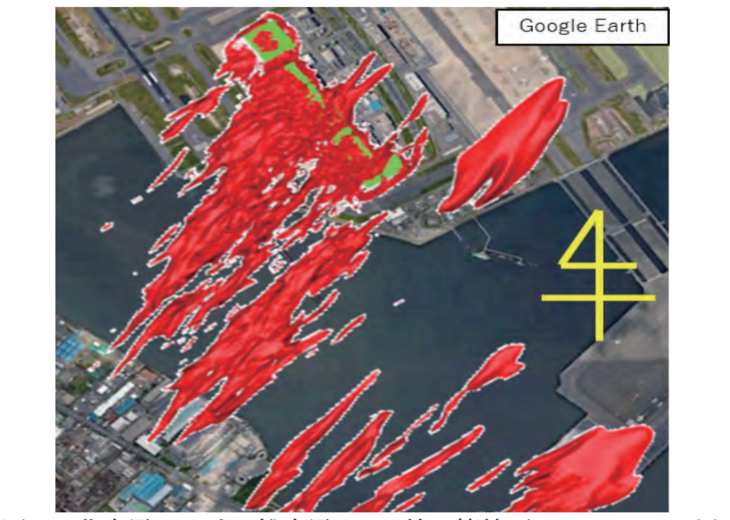
羽田空港の格納庫後流中を飛行する着陸機の安全性の研究
- 著者・発表者
- 岩渕 秀(東北大学)
焼野 藍子(東北大学)
大林 茂(東北大学)
吉村 僚一(東北大学)
菊地 亮太(DoerResearch株式会社)
- 掲載・発表場所
- ながれ (日本流体力学会誌)
- 掲載・発表日
- 2020年4月25日
空港周辺で発生する低層風擾覧が着陸時等において航空機に及ぼす危険度を評価するため流体解析を利用した手法を構築。
・羽田空港を対称に解析えお実施し,解析結果が良好で,その結果をもとに羽田空港で低層風擾覧を回避できる安全な飛行経路を提案。
・本結果は低層風擾覧を発生する空港の着陸制限に関する法規策定や運行管理者やパイロットへの指導等に貢献が可能。
・さらにフライトシミュレータに組み込み,乱気流遭遇時の航空機挙動シミュレーションができれば航空機安全性向上への貢献が可能。
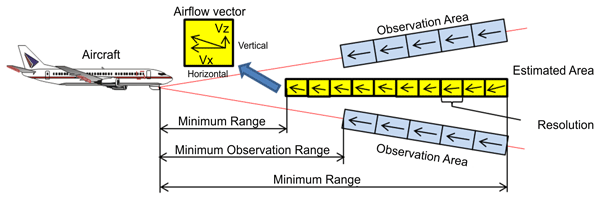
Real-time estimation of airflow vector based on lidar observations for preview control
- 著者・発表者
- Ryota Kikuchi(Japan Aerospace Exploration Agency, DoerResearch Inc.)
Takashi Misaka(National Institute of Advanced Industrial Science and Technology
Shigeru Obayashi(Tohoku University)
Hamaki Inokuchi(Japan Aerospace Exploration Agency)
- 掲載・発表場所
- Atmospheric Measurement Techniques
- 掲載・発表日
- 2020年12月4日
As part of control techniques, gust-alleviation systems using airborne Doppler lidar technology are expected to enhance aviation safety by significantly reducing the risk of turbulence-related accidents. Accurate measurement and estimation of the vertical wind velocity are very important in the successful implementation of such systems. An estimation algorithm for the airflow vector based on data from airborne lidars is proposed and investigated for preview control to prevent turbulence-induced aircraft accidents in flight. An existing technique – simple vector conversion – assumes that the wind field between the lidars is homogeneous, but this assumption fails when turbulence occurs due to a large wind-velocity fluctuation. The proposed algorithm stores the line-of-sight (LOS) wind data at every moment and uses recent and past LOS wind data to estimate the airflow vector and to extrapolate the wind field between the airborne twin lidars without the assumption of homogeneity. Two numerical experiments – using the ideal vortex model and numerical weather prediction, respectively – were conducted to evaluate the estimation performance of the proposed method. The proposed method has much better performance than simple vector conversion in both experiments, and it can estimate accurate two-dimensional wind-field distributions, unlike simple vector conversion. The estimation performance and the computational cost of the proposed method can satisfy the performance demand for preview control.
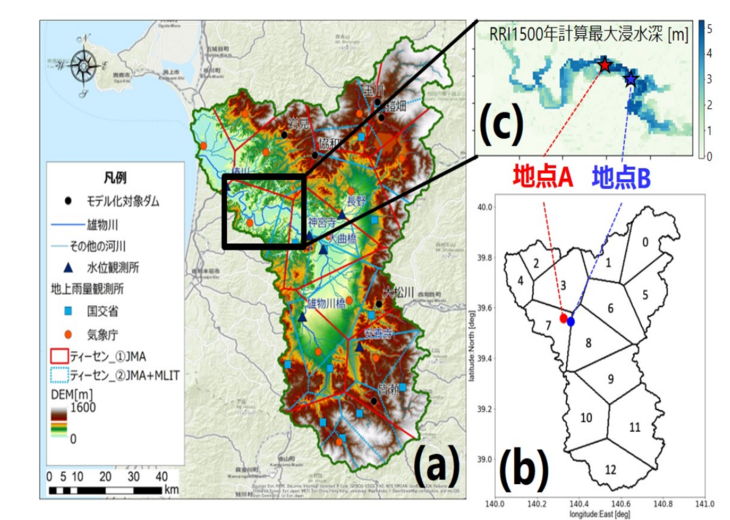
回帰学習器のアンサンブル学習による降雨洪水氾濫モデル・エミュレータ
- 著者・発表者
- 小槻 峻司(千葉大学)
桃井 裕広(DoerResearch株式会社)
菊地 亮太(京都大学)
渡部 哲史(東京大学)
山田 真史(京都大学)
阿部 紫織(三井共同建設コンサルタント株式会社)
綿貫 翔(株式会社 建設環境研究所)
- 掲載・発表場所
- 土木学会論文集BI
- 掲載・発表日
- 2020年
気象・気候分野で進められている大アンサンブル予測データの有効利用を目的に,降雨流出氾濫モデルのエミュレータを開発した。このエミュレータは,降雨の空間・時間分布から,降水イベントの最大浸水深を予測する。まず3種の正則化回帰学習器を作成し,Random Forestによるそれらのアンサンブル学習を行った。この構成は,劣決定問題における過学習回避と,弱学習器を複数とりまとめるスタッキングによる非線形変換という2つの役割を果たしている。最大浸水深の予測精度には,回帰学習器の正則化手法による違いは殆ど見られず,回帰学習器は深い最大浸水深を過小評価する傾向が見られた。複数の回帰学習器のスタッキングにより,学習器の予測精度が大幅に改善した。特に,回帰学習器に見られた最大浸水深の過小評価が大きく改善した。